Artificial Intelligence will bring fundamental changes
to team management and leadership.

“There are no bad teams, there are only bad leaders” – the famous axiom has advised many generations of leaders throughout history. Indeed, in teams where the members are assigned more or less randomly, without systematic long-term analysis of their skill match, this is still very much the case. Even today there are very few teams, be they university departments, project teams, military units or football squads, that are actually set up based on high quality data and analysis.
The reason for this is very simple: the optimal management of team composition requires inordinate amounts of time, money and data. That is, things that have been only possible to provide to a select few teams in most organizations. Your city’s hospital might have a world-famous heart-transplant team that was carefully assembled, tested and reassembled until it reached peak performance. But is the same true to the Wednesday night shift at the same hospital’s ER department? Probably not.
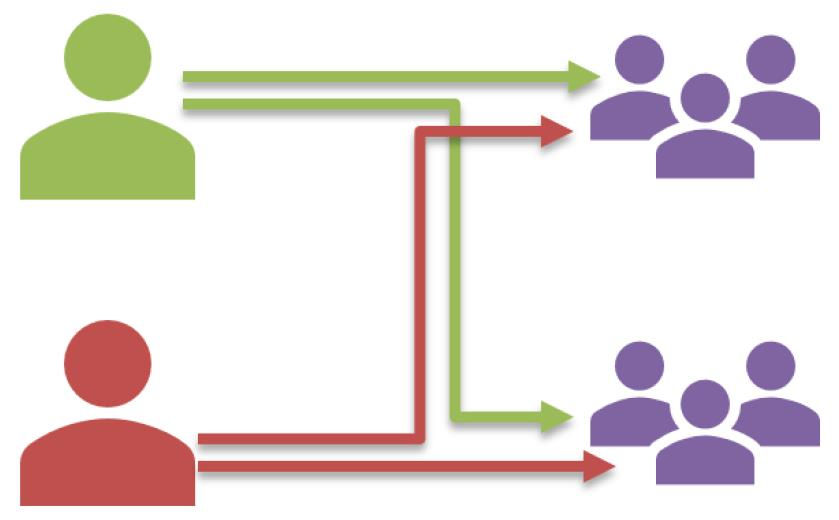
This means that up until now the axiom was true, although a more precise way to put it would be: “Most teams are not good, but they only get very bad under bad leaders.” However, this may all change with the emergence of big data and real-time team-management algorithms. What has been impossible until now, will soon become a reality: organizations will have cost effective ways to create good teams on a scale never seen before. There is a catch, however: creating good teams by definition also means that they will be creating average and outright bad teams too.
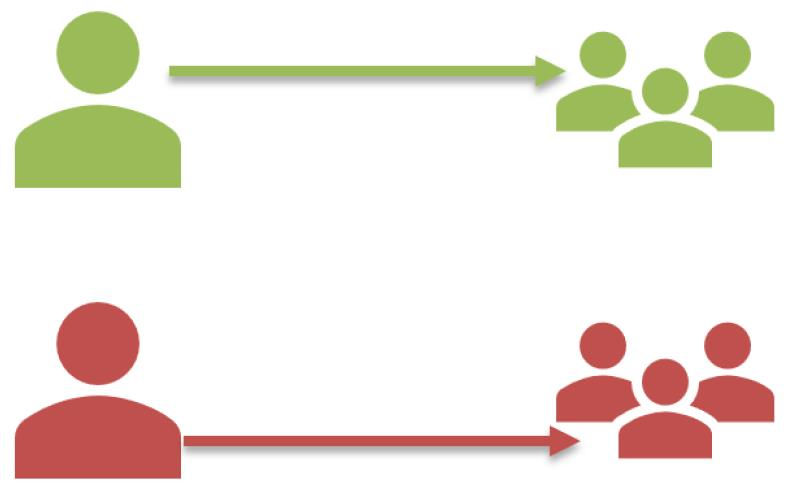
- - -
To understand how it can happen and what it may lead to, let’s look at a field where large-scale real-time team-configuration is already old news: E-Sports. Calculating the relative skill levels of players has long been a critical question in sport. The widely used Élő rating system (named after its creator Árpád Élő, a Hungarian-American physics professor), for example has been popular in competitor-versus-competitor games since the 1960’s. Similar, but more team focused rating systems (like Glicko and TrueSkill) are critical components in all E-Sports. The following example explains how the rating algorithms work in Rainbow Six Siege, a team based tactical shooter video game with a player base of 30 million:
The algorithm used in Rainbow Six Siege assigns two values to each player: an estimation of their skill (μ) and the uncertainty of this estimation (σ). The players’ skills are distributed along a bell curve, centered around 25 (High Silver – Low Gold, Figure 1).
Figure 1
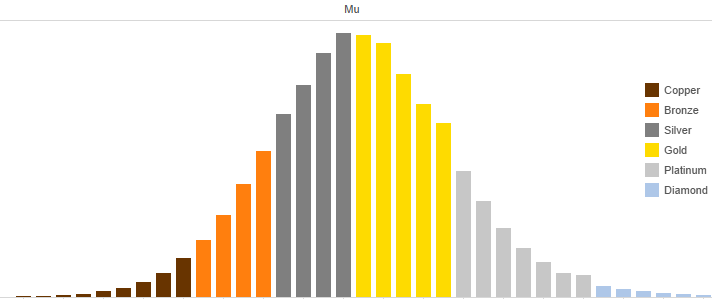
Let’s take a simple example with only two players, Craig and Justin. Justin is a Gold player (μ= 29) and has been playing at that level for quite some time. Justin’s rank has a low uncertainty value attached to it, so his σ= 3. Justin faces Craig (a Copper ranked player) and loses. Due to the low uncertainty value, it is likely that Justin just had a bad game, so his skill level would decrease only a little, resulting in μ = 28. This is due to the system taking into account the rank of the enemy he has lost to. If Justin lost to someone that was Gold, the adjustment would be closer to μ = 28.5. Losing to a Diamond would have resulted in a change to μ = 28.9.
Craig is still relatively new to the game, and thus the system is attempting to determine where he should be placed (μ= 17 and σ= 6). As Craig won the match, his skill level will increase to 20. His victory against Justin has helped the algorithm to determine that he may be a better player, so his uncertainly has dropped a small amount (σ= 5.5). The large increase in skill level rating for Craig is a result of Craig being a fairly unknown player, and the algorithm’s confidence in Justin’s placement.
One thing to note is that, in this system, the player’s personal performance doesn’t impact his rating. What the system measures is the players contribution to their team. The idea is that if they play well, and are an asset to their team, they will naturally win more matches in the long run. This positive influence on winning matches is what the rating system measures.
In E-Sports, game designers are continuously working on a system where the algorithm can more accurately predict the player’s skill and create teams with lower skill difference. While in this case the focus is on balancing the opposing teams, similar algorithms can also help HR managers and team leaders to create teams of previously unimaginable homogeneity and planned (in)efficiency. If an AI can gradually create more and more perfect balance BETWEEN teams, then it can also make better balance INSIDE them. Returning to our E-Sport example, we can see how this “gradual” process looks in practice:
The game designers continuously improve their system’s algorithm based on player feedback and game data. Figure 2 shows how Rainbow Six Siege’s ranking algorithm improved between the Dust line and Red Crow editions. Note how Red Crow’s algorithm resulted in 50% less difference in team skill compared to the earlier Dust Line version, and that the balance in Red Crow also proved to be more stable as time progressed (see the number of days on Axis X).
As Figure 3 shows, the improved team composition algorithm also led to more balanced match results. Although matches with more distinct results (4-0, 4-1) are still dominant, if we compare data between Dust Line and Red Crow, we can see that in Red Crow there were less matches with extremely one-sided results than that in Dust Line (-2.3%, -0.5%). Moreover, there were more matches with well-balanced results (4-2, 5-3, 5-4) in the later edition (+1.1%, +0.7%, +0.9%, respectively).
Figure 2
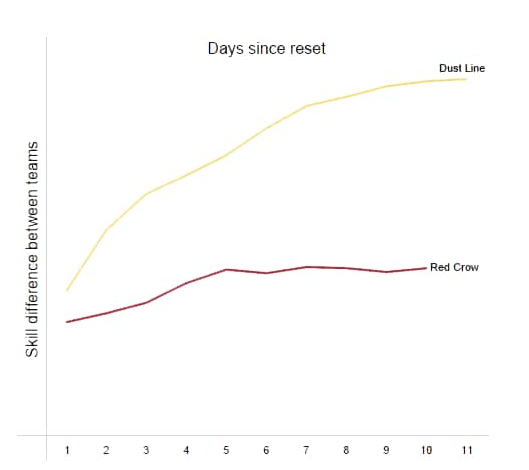
Figure 3
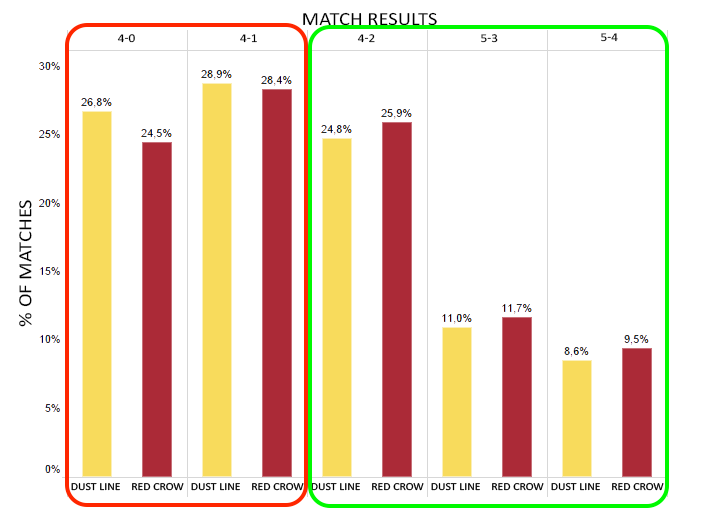
- - -
As we can see, skill or performance rating algorithms already work reasonably well in E-Sports. It may not take too long until similar systems will also become available for general use. We might soon be assigned dynamically to teams where we really fit into, by letting our professional and cooperative skills be measured and analysed in real time. Having a well-understood mix of high- and low-performing teams could lead to higher efficiencies in big organizations compared to the old patchwork of random teams. Having elite teams at hand to deal with the most difficult tasks, while letting weaker teams work on time-consuming but less-critical ones, might very well result in net gain in efficiency.
However, while we might enjoy having more flexible positions and won’t miss being trapped in a team where we carry 90% of the workload (or, conversely, where that misunderstood genius just cannot stop complaining about how bad we are), caution might be advisable before introducing such rating systems and team creation methods to HRM.
For one, randomly generated teams are not without advantages. Currently lower skilled / lower experience individuals have a fairly good chance to learn from stronger teammates and thus improve their skills. Losing that opportunity without providing enough new channels for learning may have adverse effects on both the individual employees and the organization. Furthermore, there may be serious psychological issues arising from having wide, officially recognized skill differences between teams. Being branded as a 4th or 5th rate employee does not help morale.
It is possible that there is no universal answer to these challenges. AI-based live ranking systems will most probably help many an organization to considerably raise its efficiency. But there will be ones that should keep away from these ‘great advances’ at all price. And then some may find these systems useful in a given year and then suffer from them in the next, or vice versa:
Having a high-low performance mix of elite Assault Divisions and weak Defence Divisions worked very well for the German army in the static phases of World War I. The same, however, was far from being true in the much more dynamic environment of World War 2. The creation of a high number of special units sucked out too many potential leaders and mentors from the ‘normal’ divisions, and thus resulted in a net loss of army-wide capabilities.
SoCient | STS Hub
Science, Technology & Society
Follow & let your ideas flood
Comentarios